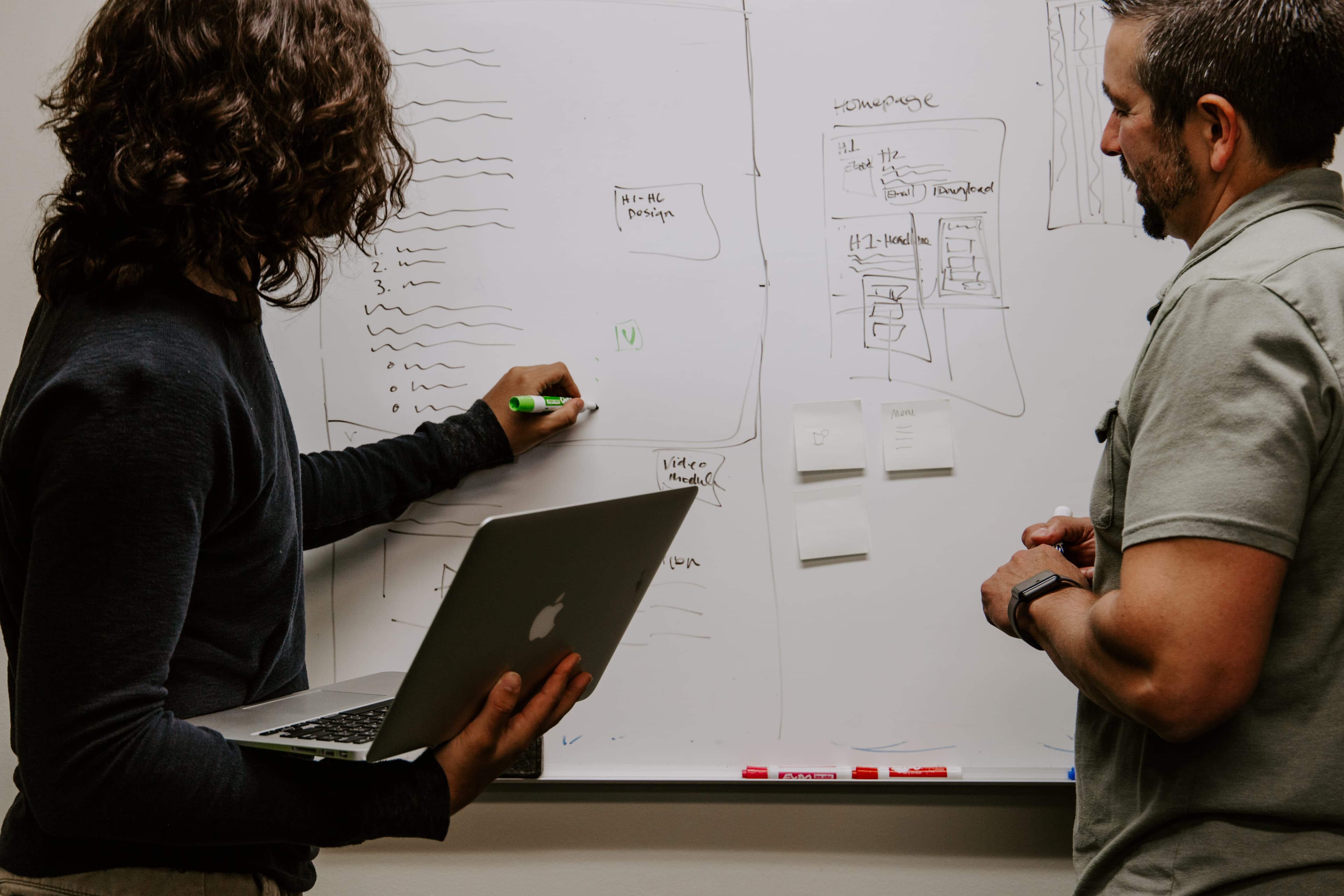
Data Analytics for Warehouse Optimization: Leveraging Insights for Performance Improvement
In today's fast-paced and competitive business world, optimizing warehouse operations has become more vital than ever. With the increasing complexity of supply chains, companies are realizing the need to harness the power of data analytics to . By leveraging data-driven approaches, businesses can gain a competitive edge by streamlining operations, reducing costs, and enhancing customer satisfaction. In this article, we will explore the various ways that data analytics can revolutionize warehouse optimization and discuss the key components, impacts, challenges, and future trends in this field.
Understanding the Role of Data Analytics in Warehouse Optimization
Before we delve into the specifics, it is essential to understand what data analytics entails in the context of warehouse management. Data analytics involves to drive informed decision-making. In the warehouse optimization context, it involves collecting, analyzing, and interpreting data from various sources to identify patterns, trends, and outliers that can significantly impact warehouse performance.
Defining Data Analytics
Data analytics is the process of examining raw data to draw conclusions and uncover hidden patterns, correlations, and trends. It involves applying statistical algorithms, machine learning techniques, and artificial intelligence to make sense of structured and unstructured data. In the context of warehouse optimization, data analytics enables businesses to gain valuable insights into their inventory, operations, and customer behavior, paving the way for proactive decision-making.
When it comes to data analytics, there are various techniques and tools that can be used. For example, businesses can employ descriptive analytics to understand what has happened in the past, diagnostic analytics to determine why certain events occurred, predictive analytics to forecast future outcomes, and prescriptive analytics to recommend the best course of action.
Furthermore, data analytics can involve the use of key performance indicators (KPIs) to measure and monitor warehouse performance. These KPIs can include metrics such as order fulfillment rate, inventory turnover, on-time delivery, and warehouse capacity utilization. By analyzing these KPIs, businesses can identify areas for improvement and take proactive measures to optimize their warehouse operations.
The Intersection of Data Analytics and Warehouse Management
Data analytics and warehouse management are not mutually exclusive; in fact, they go hand in hand. Warehouse management encompasses all the activities involved in managing the physical flow of goods within a warehouse, from receiving to storage to shipping. Utilizing data analytics enhances the efficiency and effectiveness of these processes by analyzing data and providing actionable insights to .
For example, data analytics can help businesses identify the optimal storage locations for different products based on their demand patterns. By analyzing historical sales data, businesses can determine which products are frequently ordered together and place them in close proximity to each other in the warehouse. This reduces the time and effort required to pick and pack orders, ultimately improving order fulfillment rates and customer satisfaction.
In addition, data analytics can enable businesses to forecast demand more accurately. By analyzing historical sales data, market trends, and external factors such as promotions or seasonal fluctuations, businesses can predict future demand with greater precision. This allows them to optimize inventory levels, ensuring that they have the right amount of stock on hand to meet customer demand without incurring excess carrying costs.
Moreover, data analytics can help businesses identify operational inefficiencies and bottlenecks within the warehouse. By analyzing data on order processing times, picking and packing errors, and equipment utilization, businesses can pinpoint areas that require improvement. They can then implement process changes, invest in automation technologies, or reconfigure warehouse layouts to streamline operations and increase productivity.
Overall, data analytics plays a crucial role in warehouse optimization by providing businesses with the insights they need to make informed decisions and continuously improve their operations. By leveraging the power of data, businesses can enhance efficiency, reduce costs, and deliver exceptional customer experiences in today's competitive marketplace.
Key Components of Data-Driven Warehouse Optimization
Data-driven warehouse optimization involves leveraging data analytics to improve different aspects of warehouse management. Let's explore some essential components:
Inventory Management through Data Analytics
One of the critical challenges in warehouse management is maintaining optimal inventory levels. By analyzing historical sales data, customer buying patterns, and market trends, data analytics enables businesses to predict demand more accurately. This helps in optimizing inventory levels, reducing stockouts, and minimizing carrying costs.
For example, let's consider a retail company that sells clothing. By analyzing data on customer preferences, such as popular sizes, colors, and styles, the company can ensure that it has sufficient stock of the most in-demand items. This not only improves customer satisfaction but also reduces the risk of losing sales due to out-of-stock items.
Data analytics can also help identify slow-moving or obsolete inventory. By analyzing sales trends and customer demand, businesses can make informed decisions about discounting or liquidating such inventory, freeing up valuable warehouse space and capital.
Predictive Analytics for Demand Forecasting
Predictive analytics plays a vital role in demand forecasting, enabling businesses to anticipate customer demand and plan their inventory accordingly. By analyzing historical data, market trends, and external factors such as promotional activities or seasonal variations, businesses can proactively adjust their inventory levels, reducing the risk of overstocking or understocking.
For instance, a grocery store can use predictive analytics to forecast demand for perishable items like fruits and vegetables. By considering factors like weather patterns, upcoming holidays, and past sales data, the store can ensure that it has the right quantity of fresh produce available to meet customer demand. This not only minimizes wastage but also maximizes sales opportunities.
Predictive analytics can also help businesses optimize their supply chain by identifying potential disruptions or bottlenecks. By analyzing data on supplier performance, transportation routes, and lead times, businesses can make informed decisions about sourcing, inventory replenishment, and distribution, ensuring smooth operations and timely delivery.
Enhancing Warehouse Operations with Real-Time Data
Real-time data collection and analysis have revolutionized warehouse operations. By integrating advanced technologies such as Internet of Things (IoT) devices and sensors, businesses can capture real-time data on inventory levels, equipment performance, and employee productivity. This data allows for timely decision-making, optimizing resource allocation, and reducing operational bottlenecks.
For example, RFID tags can be used to track the location and movement of inventory within the warehouse. This real-time data enables businesses to optimize their picking and packing processes, reducing errors and improving efficiency. Additionally, sensors can monitor equipment health and performance, allowing for proactive maintenance and minimizing downtime.
Real-time data also enables businesses to monitor employee productivity and identify areas for improvement. By analyzing data on pick rates, order accuracy, and time spent on different tasks, businesses can identify training needs, optimize workflows, and incentivize high performance.
In conclusion, data-driven warehouse optimization offers numerous benefits for businesses. By leveraging data analytics, businesses can improve inventory management, enhance demand forecasting, and optimize warehouse operations. This not only leads to cost savings and efficiency gains but also improves customer satisfaction and competitive advantage in the market.
The Impact of Data Analytics on Warehouse Performance
Data analytics has a transformative effect on warehouse performance, influencing several key areas:
Improving Efficiency and Productivity
By leveraging data analytics, businesses can identify inefficiencies in warehouse processes and take corrective actions. For example, analyzing equipment performance data can help identify bottlenecks or maintenance requirements, optimizing equipment utilization and reducing downtime. Additionally, data analytics can provide insights into employee productivity, enabling businesses to streamline workflows and allocate resources effectively.
Furthermore, data analytics can help businesses identify patterns and trends in order fulfillment, allowing them to optimize inventory management. By analyzing historical data on order volume, seasonal fluctuations, and customer preferences, businesses can make informed decisions about inventory levels, reducing the risk of stockouts or excess inventory. This not only improves efficiency but also minimizes costs associated with inventory holding and storage.
Reducing Operational Costs
Warehouse operations come with substantial costs, from labor to inventory holding to energy consumption. Data analytics helps identify areas of cost inefficiencies, such as excessive storage space utilization or inefficient picking routes. By optimizing these processes using data-driven insights, businesses can reduce operational costs, enhance profitability, and gain a competitive advantage.
Moreover, data analytics can assist in optimizing labor utilization. By analyzing data on employee performance, businesses can identify training needs, implement performance-based incentives, and improve workforce planning. This not only reduces labor costs but also enhances employee satisfaction and retention.
Enhancing Customer Satisfaction
Delivering an exceptional customer experience is crucial in today's competitive marketplace. Data analytics allows businesses to gain insights into customer behavior, preferences, and satisfaction levels. By understanding these insights, businesses can tailor their warehouse operations to meet customer expectations, ensuring accurate and timely order fulfillment, minimizing errors, and improving overall customer satisfaction.
In addition, data analytics can enable businesses to implement predictive analytics models to forecast customer demand. By analyzing historical sales data, market trends, and external factors, businesses can anticipate customer needs and adjust their inventory levels and order fulfillment processes accordingly. This proactive approach not only improves customer satisfaction but also reduces the risk of stockouts and improves overall supply chain efficiency.
Furthermore, data analytics can help businesses identify opportunities for personalization and customization. By analyzing customer data, businesses can identify individual preferences and tailor their product offerings or promotional strategies to specific customer segments. This targeted approach enhances customer satisfaction and loyalty, leading to increased sales and revenue.
Overcoming Challenges in Implementing Data Analytics in Warehouses
While the benefits of data analytics in warehouse optimization are undeniable, implementing and leveraging data analytics can present various challenges:
Data Quality and Integration Issues
The quality and accuracy of data play a vital role in deriving meaningful insights. Inaccurate or incomplete data can lead to flawed analyses and poor decision-making. Additionally, integrating data from disparate sources, such as inventory management systems or enterprise resource planning (ERP) systems, can be complex and time-consuming. Overcoming these challenges requires robust data collection processes, data cleansing techniques, and integration strategies.
One of the key aspects of ensuring data quality is establishing data governance practices. This involves defining data standards, implementing data validation rules, and conducting regular data audits. By enforcing data governance, warehouses can maintain high-quality data, which in turn enhances the accuracy and reliability of analytics results.
Another challenge in data integration is dealing with data silos. Warehouses often have multiple systems that store data independently, making it difficult to consolidate and analyze information holistically. To overcome this, organizations can invest in data integration tools and technologies that enable seamless data flow between different systems. This integration not only improves data accuracy but also enhances the efficiency of warehouse operations.
Skills and Training for Data-Driven Warehouse Management
Implementing data analytics in warehouses requires personnel with the right skill set and expertise. Data scientists, analysts, and warehouse managers must have a solid understanding of data analytics techniques, statistical modeling, data visualization, and the ability to translate data into actionable insights. Providing adequate training and upskilling opportunities is crucial to harnessing the full potential of data analytics in warehouse optimization.
Organizations can invest in training programs and workshops to enhance the data analytics skills of their workforce. These programs can cover topics such as data collection methods, data preprocessing techniques, advanced analytics algorithms, and data visualization tools. By equipping employees with the necessary skills, warehouses can empower their workforce to make data-driven decisions and optimize warehouse operations.
In addition to training, fostering a data-driven culture within the organization is essential. This involves promoting the use of data analytics in decision-making processes and encouraging employees to explore and experiment with data. By creating a culture that values data-driven insights, warehouses can drive continuous improvement and innovation in their operations.
Future Trends in Data Analytics for Warehouse Optimization
As technology continues to evolve, so do the trends in data analytics for warehouse optimization. Here are a few noteworthy trends to watch:
The Rise of AI and Machine Learning in Warehouse Management
Artificial intelligence (AI) and machine learning (ML) are becoming increasingly prevalent in warehouse management. These technologies enable predictive analytics, anomaly detection, and intelligent automation, paving the way for more efficient and autonomous warehouse operations. AI-powered algorithms can optimize processes like inventory replenishment, demand forecasting, and route optimization, revolutionizing warehouse management.
The Role of IoT in Real-Time Data Collection
The Internet of Things (IoT) is transforming warehouse operations by enabling real-time data collection through interconnected devices and sensors. IoT devices can monitor temperature, humidity, inventory levels, and equipment performance, providing valuable insights into the overall warehouse ecosystem. Real-time data analytics enables proactive decision-making, predictive maintenance, and increased operational agility.
The Impact of Big Data on Warehouse Optimization
The volume, variety, and velocity of data generated by warehouses are continuously increasing. Big data technologies and analytics enable businesses to process and analyze this vast amount of data, uncovering valuable insights that were previously inaccessible. By leveraging big data analytics, warehouses can optimize operations, improve forecasting accuracy, and drive continuous improvement.
In conclusion, data analytics has emerged as a game-changer in warehouse optimization. By harnessing the power of data-driven insights, businesses can improve efficiency, reduce costs, and enhance customer satisfaction. However, implementing data analytics in warehouses may present challenges that need to be carefully addressed for successful outcomes. As technology continues to advance, future trends such as AI, IoT, and big data analytics will shape the warehouse optimization landscape. Embracing these trends will enable businesses to stay competitive and thrive in an increasingly data-driven world.
Nitro Logistics Team
Logistics Expert
The Nitro Logistics team brings together decades of experience in logistics, fulfillment, and supply chain optimization.
Stay Updated
Get the latest insights in logistics and fulfillment delivered to your inbox.